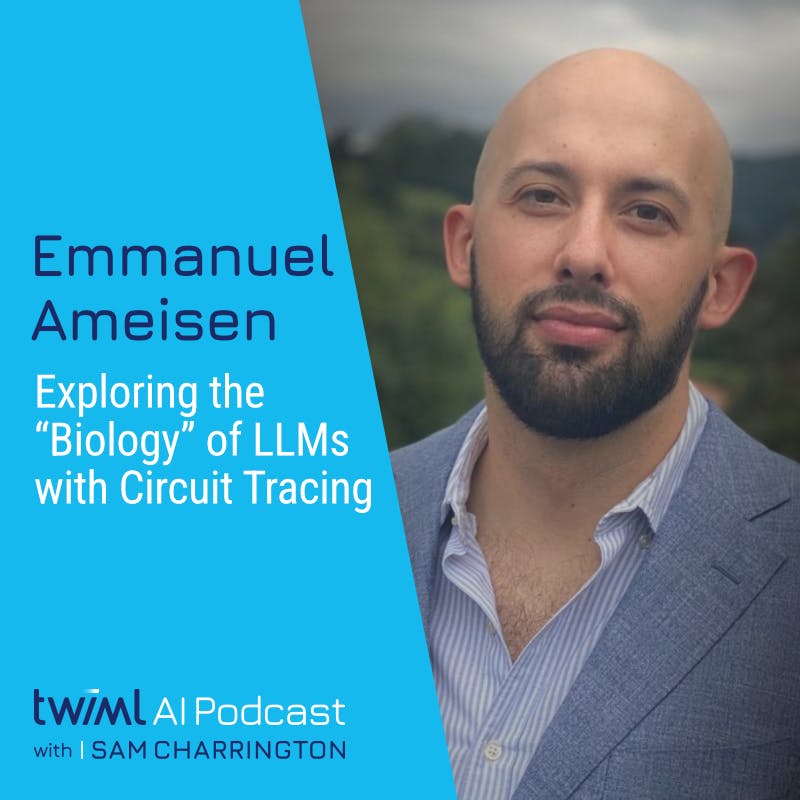
Exploring the Biology of LLMs with Circuit Tracing with Emmanuel Ameisen - #727
-
The podcast discusses mechanistic interpretability, emphasizing the importance of understanding the internal workings of large language models (LLMs) like Claude, which are often perceived as "stochastic parrots" but exhibit complex behaviors, such as planning and reasoning with linguistic tasks.
-
A key finding involves circuit tracing to analyze the pathways in which models generate responses, enabling researchers to identify the mechanisms at play and how features interact within the model, thereby revealing surprising insights about model behavior and decision-making.
-
The conversation highlights the limitations of LLMs, such as the challenges of attention mechanisms versus MLPs, and addresses the phenomenon of hallucination where models confidently provide incorrect answers, indicating a disconnection between the model's ability to generate text and factual knowledge management.
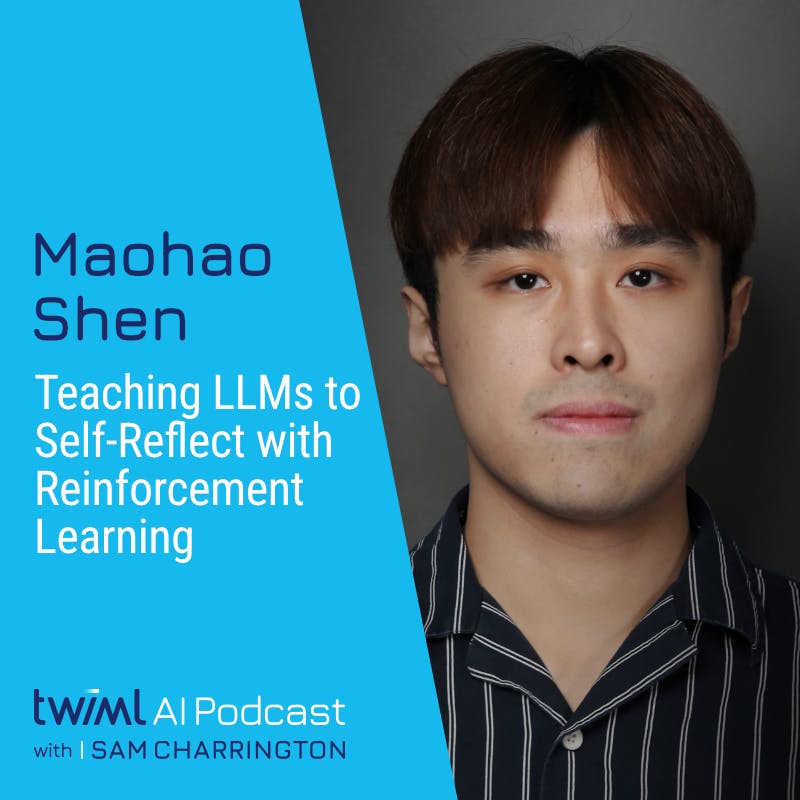
Teaching LLMs to Self-Reflect with Reinforcement Learning with Maohao Shen - #726
- Ma Shen's research focuses on enhancing AI systems by making them more intelligent and reliable, particularly through quantifying uncertainty and addressing challenges like hallucination in language models.
- The project Satori introduces a novel method of applying reinforcement learning for reasoning in language models, allowing them to self-correct and reflect on their generated responses, akin to human problem-solving processes.
- Satori demonstrates promising capabilities in both math problem-solving and general reasoning tasks, outperforming traditional instruction-based models while using significantly less training data, indicating its potential for broader application across various domains.
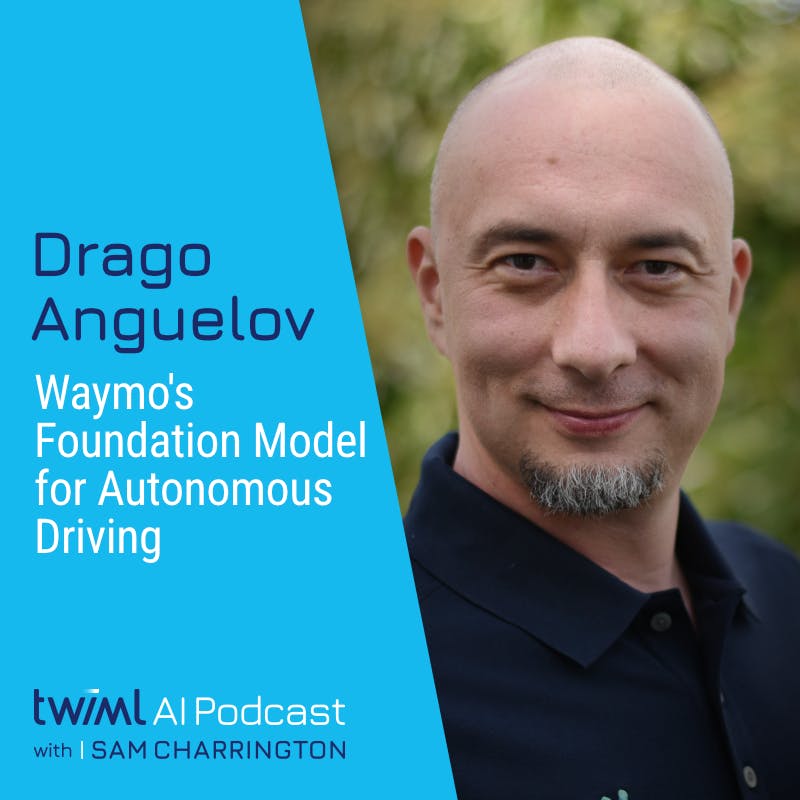
Waymo's Foundation Model for Autonomous Driving with Drago Anguelov - #725
- The integration of Foundation Models into autonomous vehicle systems is enhancing the vehicles' ability to understand complex driving scenarios, utilizing advanced techniques like Vision Language Models for improved spatial awareness and reasoning over time.
- Waymo has achieved significant growth in operational scale, now offering over 200,000 fully autonomous rides weekly across four major cities, demonstrating the potential impact of autonomous vehicles on daily transportation.
- The ongoing development of a Foundation Model tailored for autonomous driving aims to leverage extensive data and scaling techniques to improve driving generalization and predictability in diverse environments, addressing common challenges in safety and performance.
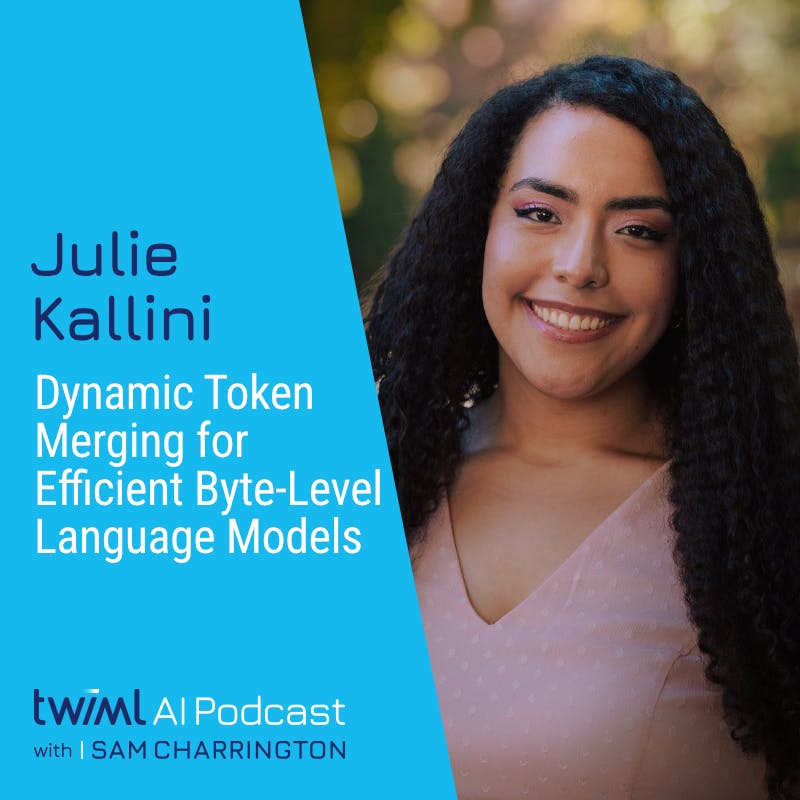
Dynamic Token Merging for Efficient Byte-level Language Models with Julie Kallini - #724
-
Tokenization is crucial for language models but inherently flawed due to language-specific compression rates, leading to inefficiencies and potential overcharging for users of under-resourced languages.
-
Julie Kini's research introduces Mr T5, a bite-level model architecture that aims to improve efficiency by using dynamic token merging, outperforming traditional token-based models in various multilingual tasks while maintaining performance.
-
The Mission Impossible paper explores the limitations of language models in learning "impossible languages," demonstrating that architectures trained in English contexts may bias performance towards natural languages, prompting further research on cognitive-linguistic alignment in AI models.
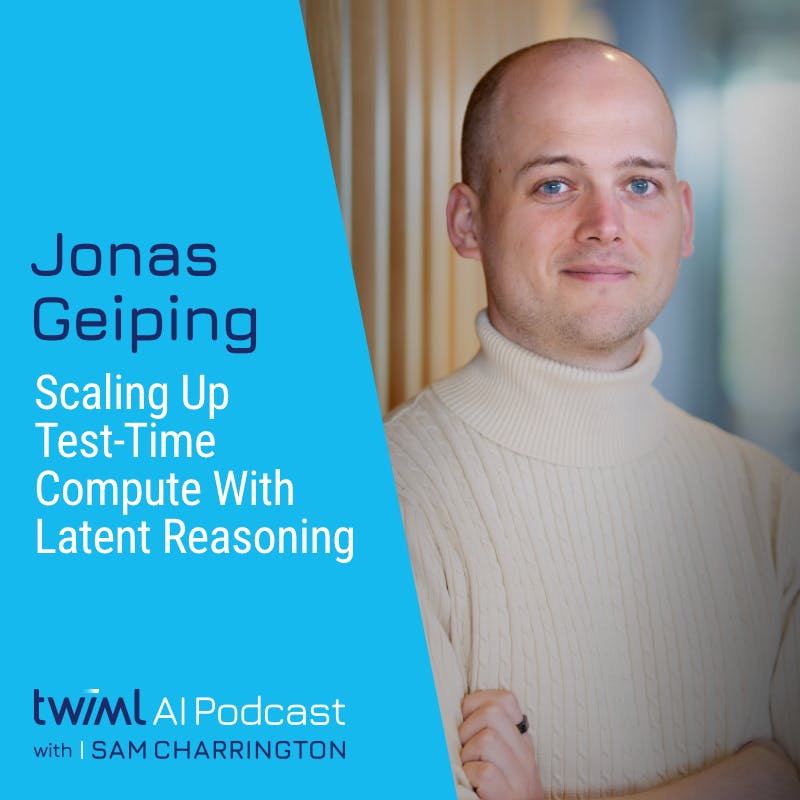
Scaling Up Test-Time Compute with Latent Reasoning with Jonas Geiping - #723
-
The podcast features a discussion on latent reasoning and recurrent depth, highlighting a novel approach to model training that allows for greater scalability and algorithm learning compared to fixed-depth architectures.
-
The conversation emphasizes the model's performance in grade school math and coding tasks, demonstrating significant improvements over traditional models with the same number of parameters by leveraging a recurrent architecture for deeper computations.
-
The speakers address the implications of this approach for model safety and understanding, suggesting that thinking internally without verbalization may provide more efficient reasoning processes while still enabling transparency in model development through open-source practices.
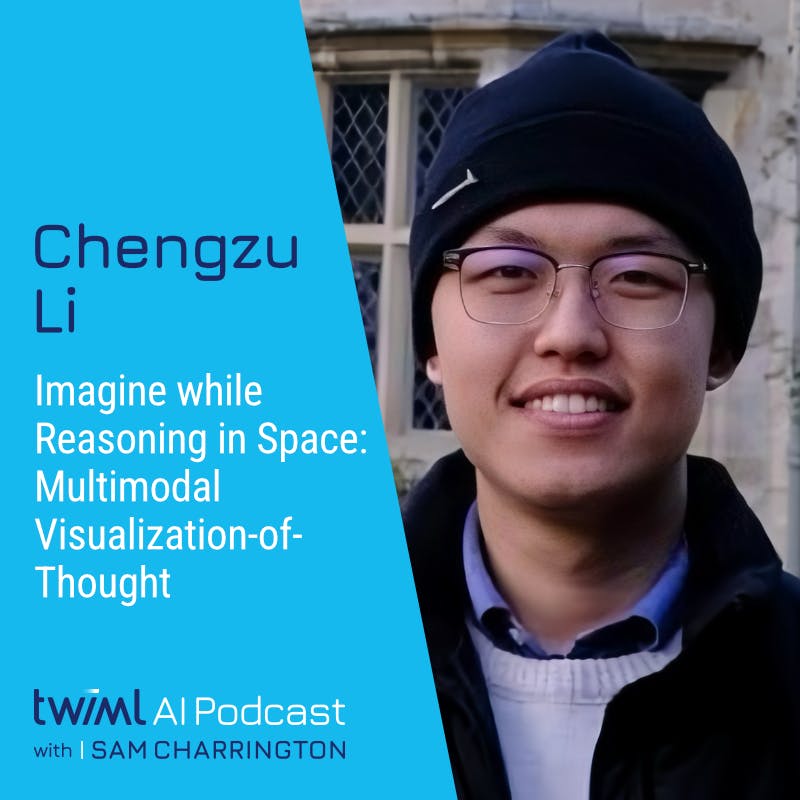
Imagine while Reasoning in Space: Multimodal Visualization-of-Thought with Chengzu Li - #722
- Chungu Lee's research focuses on multimodal reasoning and spatial reasoning, aiming to improve model performance in understanding and interacting with complex visual data as demonstrated in his recent work on navigation tasks.
- The introduction of visualization of thought (MVOT) integrates both verbal and visual reasoning, enhancing the model's ability to independently process images and text, which proves more effective in spatial tasks compared to traditional language-only models.
- Lee highlights the significance of token discrepancy loss in aligning visual and textual outputs, ensuring that generated visualizations accurately reflect intended meanings and improving overall model performance in multimodal reasoning tasks.
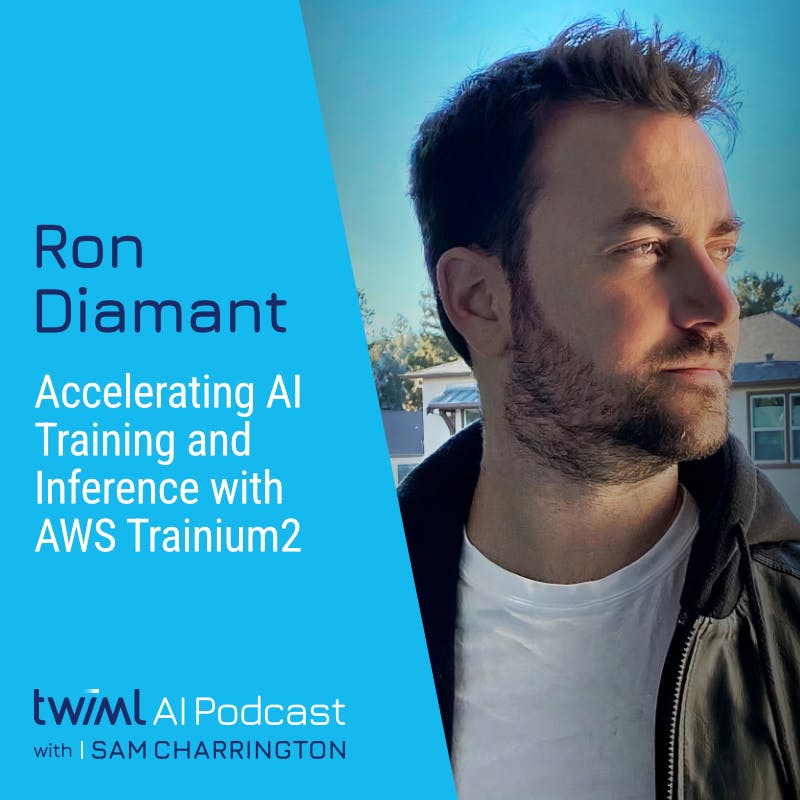
Accelerating AI Training and Inference with AWS Trainium2 with Ron Diamant - #720
- Anthropic is collaborating with AWS to build a "gigantic training cluster" with hundreds of thousands of training devices, significantly larger than its previous clusters, aimed at training the largest and most advanced frontier model.
- The Tranium architecture from AWS optimizes AI/ML workloads through a unique combination of high-performance cores and additional features like 4 to 16 sparsity, which enhances efficiency in both training and inference tasks.
- AWS's Neuron Kernel Interface (NKI) allows expert users to maximize performance by giving them low-level access to hardware, enabling them to optimize specific operations and extract the full benefits of the Tranium architecture.
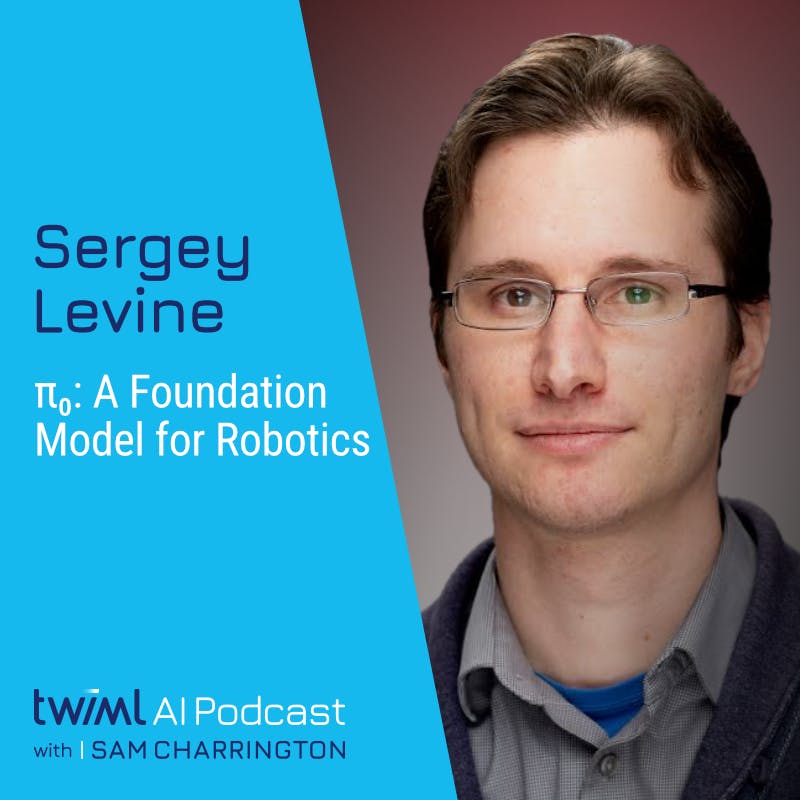
π0: A Foundation Model for Robotics with Sergey Levine - #719
- Physical intelligence aims to develop general-purpose robotic foundation models that can be adapted across a wide range of applications, which would reduce the need for starting new companies or research projects for each specific application.
- A key challenge in robotics has been the lack of large, diverse datasets, making it hard to apply machine learning effectively; however, recent advances are allowing for more transferable models that can efficiently handle various robotic tasks.
- The Pi Zero model leverages a unique pre-training and post-training methodology with an emphasis on data diversity, showing that a blend of high-quality and lower-quality data can improve the robot’s ability to handle unexpected situations and generalize better.